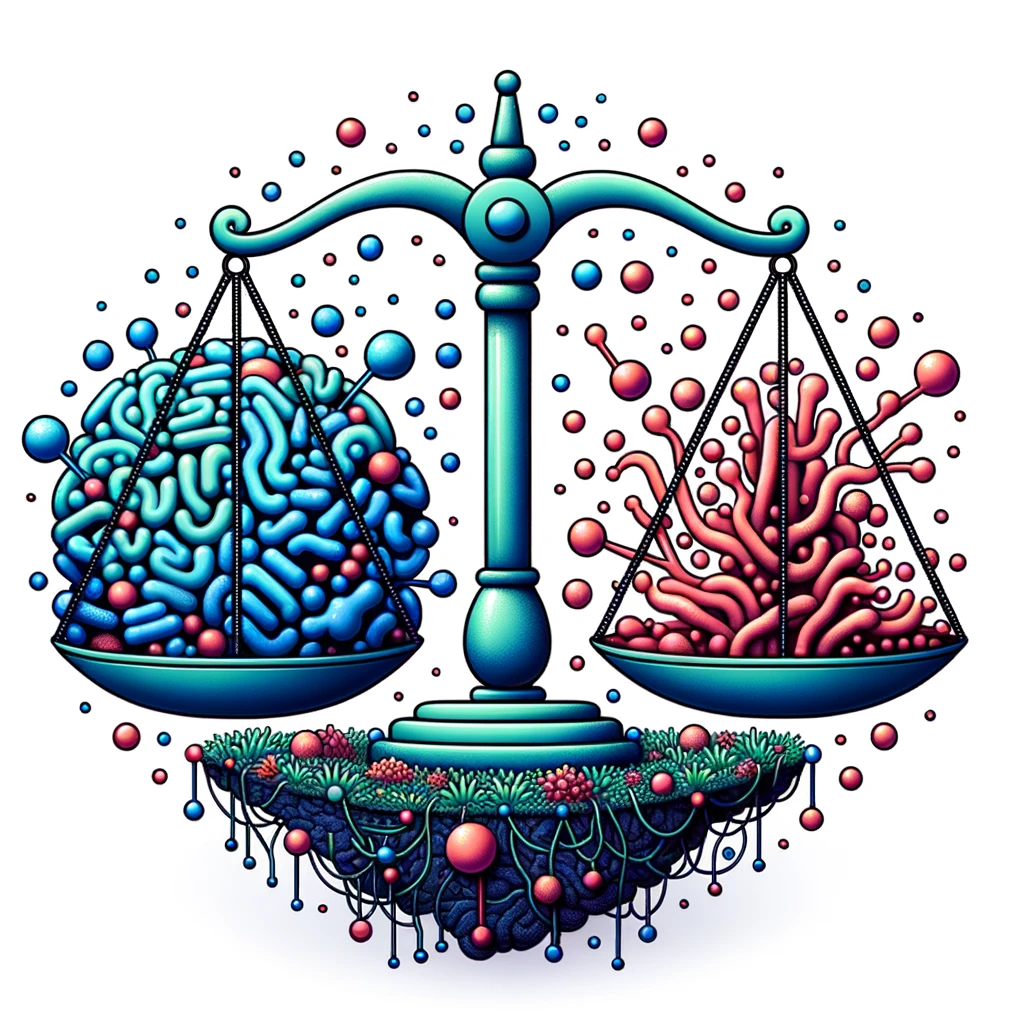
Get objects needed for a merged visualization task combining different experiments from different datasets (different X and y)
getImportanceFeaturesFBMobjects.Rd
Here we get the 4 datasets from a given prediction experiment (clf object + X + y) needed for subsequent combination with other predition experiments for combined visualization (feature prevalence in FBM + feature importance + featureEffSizes + feature prevalence in groups)
Usage
getImportanceFeaturesFBMobjects(
clf_res,
X,
y,
verbose = TRUE,
filter.cv.prev = 0.25,
scaled.importance = FALSE,
k_penalty = 0.75/100,
k_max = 0
)
Arguments
- clf_res
The result of a single experiment
- X
The feature table used as input of fit function behind experiments in clf_res
- y
The target class (binary/continuous)
- verbose
print out informaiton
- filter.cv.prev
keep only features found in at least (default: 0.25, i.e 25 percent) of the cross validation experiments
- scaled.importance
the scaled importance is the importance multipied by the prevalence in the folds. If (default = TRUE) this will be used, the mean mda will be scaled by the prevalence of the feature in the folds and ordered subsequently
- k_penalty
the sparsity penalty needed to select the best models of the population (default:0.75/100).
- k_max
select the best population below a given threshold. If (default:0) no selection is performed.